Bayesian Least-Squares Supertrees (BLeSS): a flexible method for inferring large time-calibrated phylogenies
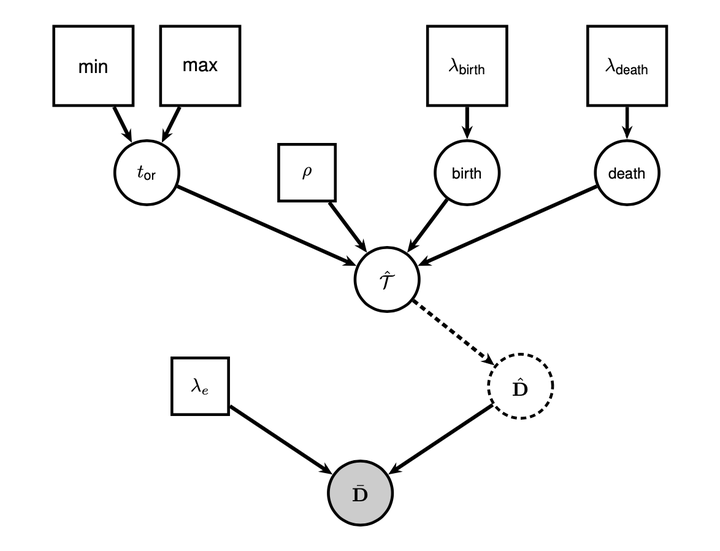
Abstract
In recent years, sparse molecular supermatrices have been used to infer time-scaled "macrophylogenies" with thousands or tens of thousands of tips. However, since the joint Bayesian inference of tree topology and divergence times remains unfeasible at such scales, tree size often comes at the cost of methodological sophistication -- a problem that has not been fully resolved by the recently introduced "backbone-and-patch" approach. Historically, supertree inference has represented a popular alternative to supermatrix-based approaches, but few supertree methods can simultaneously estimate tree topology and branch lengths, or propagate the uncertainty associated with the source trees through the estimation process. Here, we present a new method, Bayesian Least-Squares Supertrees (BLeSS), that achieves these desirable properties by combining the previously proposed average distance matrix and exponential error approaches into a single probabilistic model. The method takes a profile of ultrametric time trees as its input, and returns a posterior distribution of time-scaled supertrees as its output. BLeSS is implemented in RevBayes, and can be readily combined with other sources of information such as node calibrations, topological constraints, or differential weighting of source trees. Large-scale simulations suggest that the approach performs well across a wide range of tree shapes and missing data distributions. The approach can be extended to trees with non-contemporaneous tips by relaxing the ultrametricity assumption, potentially enabling the inference of fossil phylogenies of previously unparalleled size.